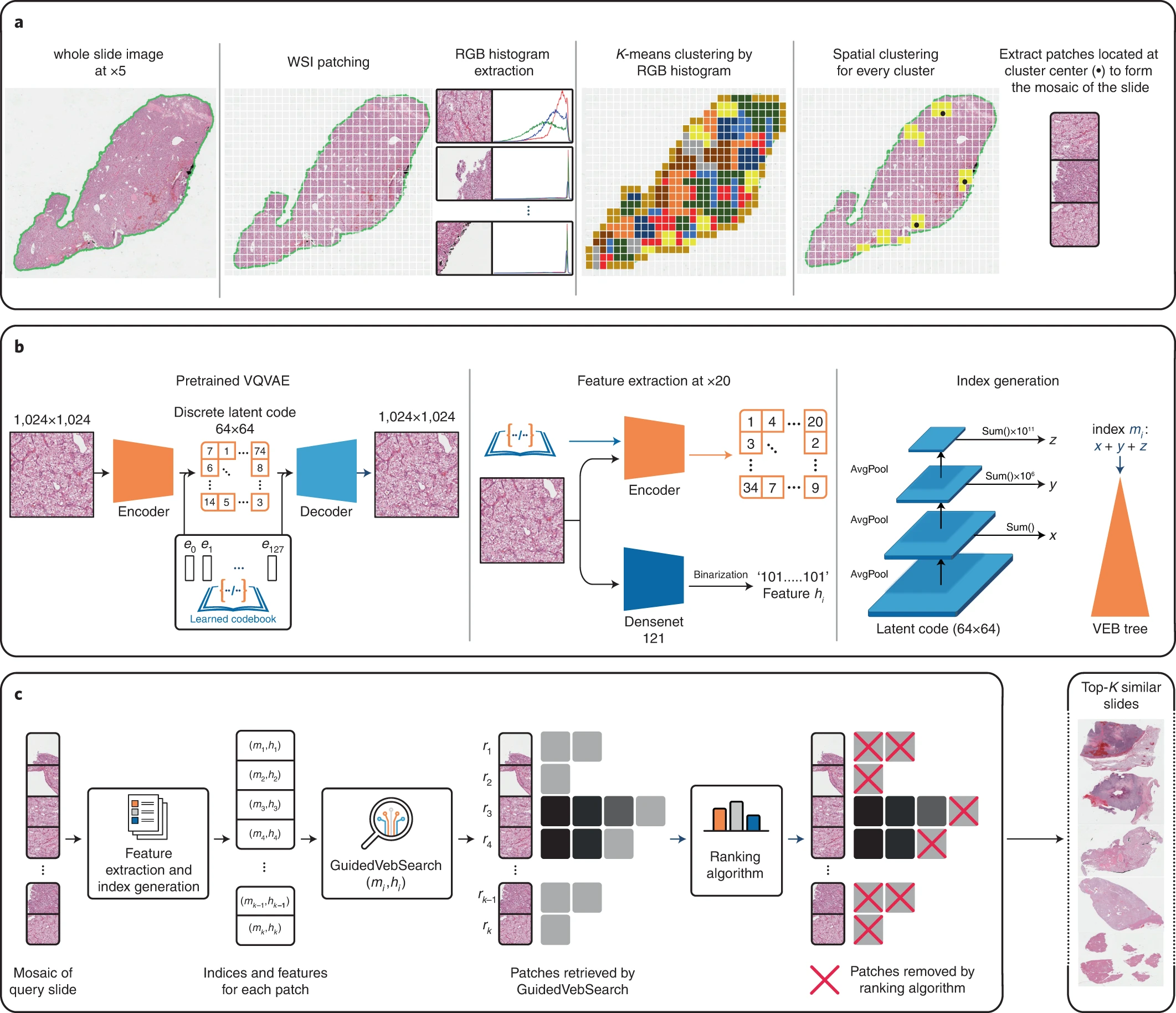
Rare diseases are often difficult to diagnose and predicting the best course of treatment can be challenging for clinicians. Investigators from the Mahmood Lab at Brigham and Women’s Hospital, a founding member of the Mass General Brigham healthcare system, have developed a deep learning algorithm that can teach itself to learn features which can then be used to find similar cases in large pathology image repositories. Known as SISH (Self-Supervised Image search for Histology), the new tool acts like a search engine for pathology images and has many potential applications, including identifying rare diseases and helping clinicians determine which patients are likely to respond to similar therapies. A paper introducing the self-teaching algorithm is published in Nature Biomedical Engineering.
“We show that our system can assist with the diagnosis of rare diseases and find cases with similar morphologic patterns without the need for manual annotations, and large datasets for supervised training,” said senior author Faisal Mahmood, PhD, in the Brigham’s Department of Pathology. “This system has the potential to improve pathology training, disease subtyping, tumor identification, and rare morphology identification.”
Modern electronic databases can store an immense amount of digital records and reference images, particularly in pathology through whole slide images (WSIs). However, the gigapixel size of each individual WSI and the ever-increasing number of images in large repositories, means that search and retrieval of WSIs can be slow and complicated. As a result, scalability remains a pertinent roadblock for efficient use.
To solve this issue, researchers at the Brigham developed SISH, which teaches itself to learn feature representations which can be used to find cases with analogous features in pathology at a constant speed regardless of the size of the database.
In their study, the researchers tested the speed and ability of SISH to retrieve interpretable disease subtype information for common and rare cancers. The algorithm successfully retrieved images with speed and accuracy from a database of tens of thousands of whole slide images from over 22,000 patient cases, with over 50 different disease types and over a dozen anatomical sites. The speed of retrieval outperformed other methods in many scenarios, including disease subtype retrieval, particularly as the image database size scaled into the thousands of images. Even while the repositories expanded in size, SISH was still able to maintain a constant search speed.
The algorithm, however, has some limitations including a large memory requirement, limited context awareness within large tissue slides and the fact that it is limited to a single imaging modality.
Overall, the algorithm demonstrated the ability to efficiently retrieve images independent of repository size and in diverse datasets. It also demonstrated proficiency in diagnosis of rare disease types and the ability to serve as a search engine to recognize certain regions of images that may be relevant for diagnosis. This work may greatly inform future disease diagnosis, prognosis, and analysis.
“As the sizes of image databases continue to grow, we hope that SISH will be useful in making identification of diseases easier,” said Mahmood. “We believe one important future direction in this area is multimodal case retrieval which involves jointly using pathology, radiology, genomic and electronic medical record data to find similar patient cases.”