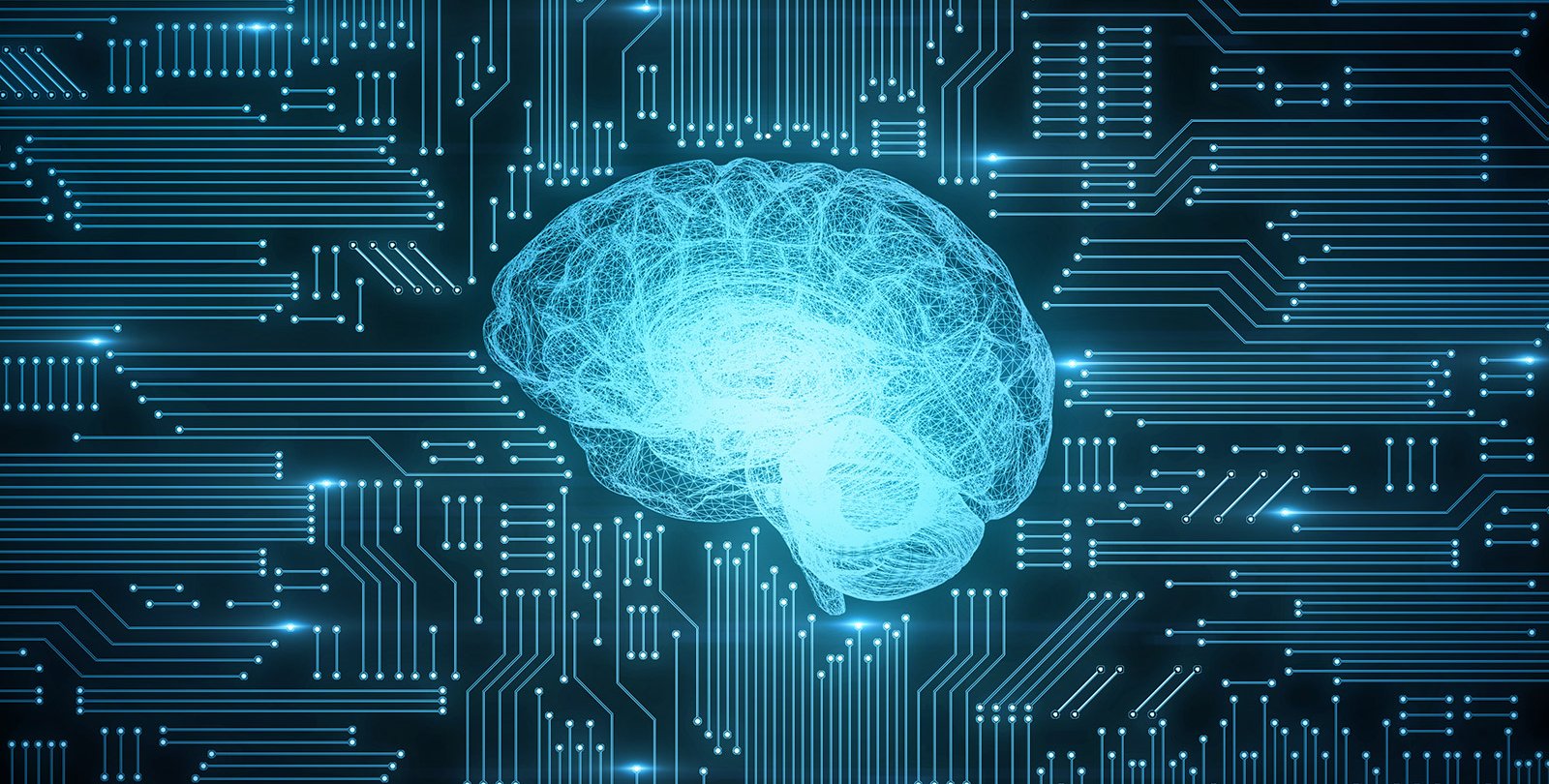
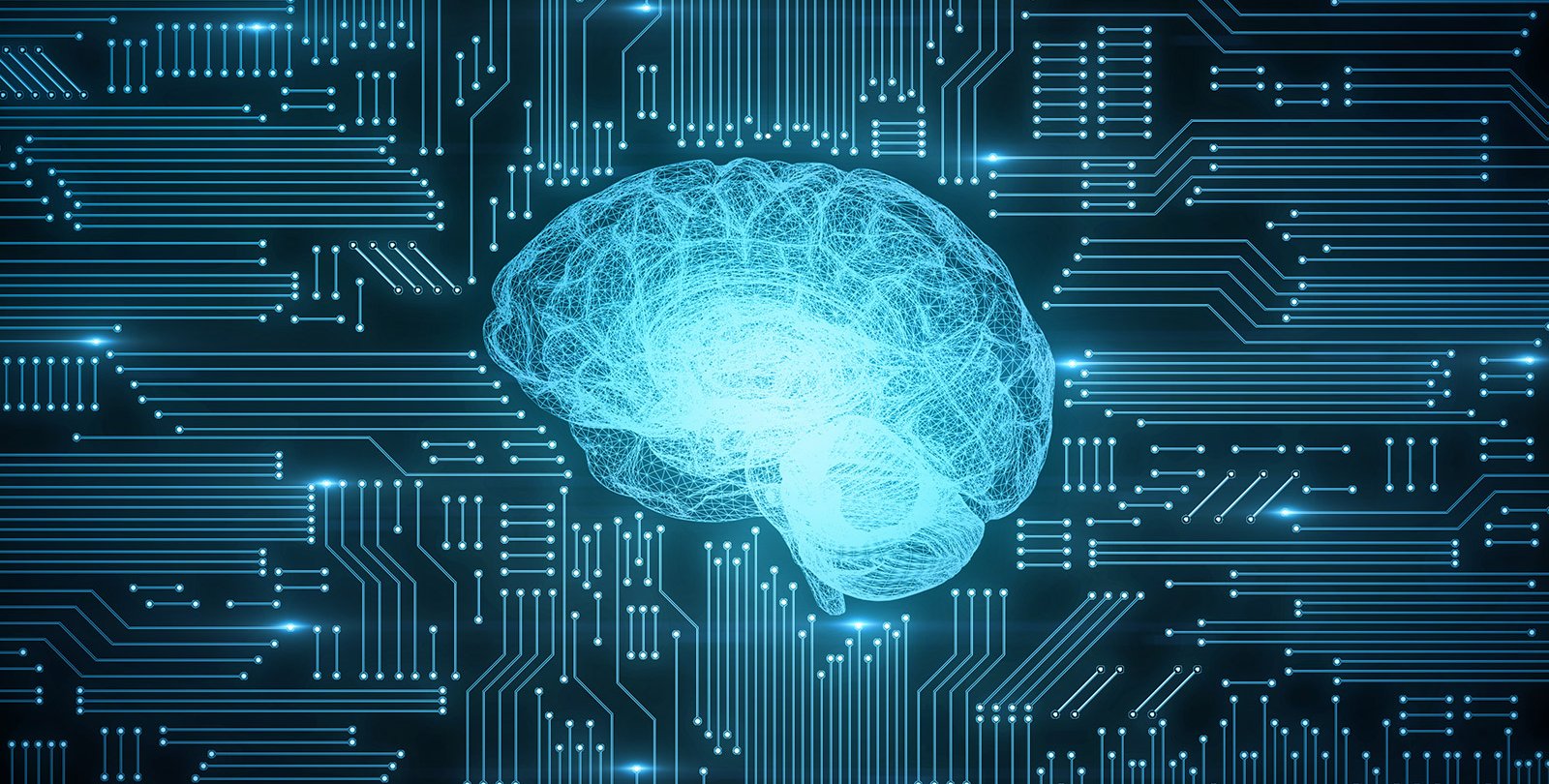
New Caltech research is showing how devices implanted into people’s brains, called brain-machine interfaces (BMIs), could one day help patients who have lost their ability to speak. In a new study presented at the 2022 Society for Neuroscience conference in San Diego, the researchers demonstrated that they could use a BMI to accurately predict which words a tetraplegic participant was simply thinking and not speaking or miming.
“You may already have seen videos of people with tetraplegia using BMIs to control robotic arms and hands, for example to grab a bottle and to drink from it or to eat a piece of chocolate,” says Sarah Wandelt, a Caltech graduate student in the lab of Richard Andersen, James G. Boswell Professor of Neuroscience and director of the Tianqiao and Chrissy Chen Brain-Machine Interface Center at Caltech.
“These new results are promising in the areas of language and communication. We used a BMI to reconstruct speech,” says Wandelt, who presented the results at the conference on November 13.
Previous studies have had some success at predicting participants’ speech by analyzing brain signals recorded from motor areas when a participant whispered or mimed words. But predicting what somebody is thinking, internal dialogue, is much more difficult, as it does not involve any movement, explains Wandelt. “In the past, algorithms that tried to predict internal speech have only been able to predict three or four words and with low accuracy or not in real time,” Wandelt says.
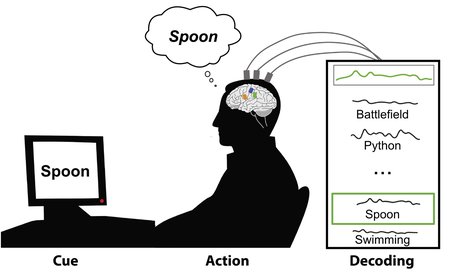
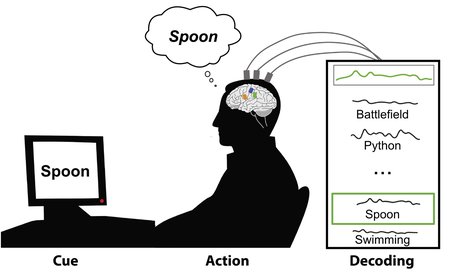
The new research is the most accurate yet at predicting internal words. In this case, brain signals were recorded from single neurons in a brain area called the supramarginal gyrus, located in the posterior parietal cortex. The researchers had found in a previous study that this brain area represents spoken words.
Now, the team has extended its findings to internal speech. In the study, the researchers first trained the BMI device to recognize the brain patterns produced when certain words were spoken internally, or thought, by the tetraplegic participant. This training period took about 15 minutes. They then flashed a word on a screen and asked the participant to say the word internally. The results showed that the BMI algorithms were able to predict eight words with an accuracy up to 91 percent.
The work is still preliminary but could help patients with brain injuries, paralysis, or diseases such as amyotrophic lateral sclerosis (ALS) that affect speech. “Neurological disorders can lead to complete paralysis of voluntary muscles, resulting in patients being unable to speak or move, but they are still able to think and reason. For that population, an internal speech BMI would be incredibly helpful,” Wandelt says.
“We have previously shown that we can decode imagined hand shapes for grasping from the human supramarginal gyrus,” says Andersen. “Being able to also decode speech from this area suggests that one implant can recover two important human abilities: grasping and speech.”
The researchers also point out that the BMIs cannot be used to read people’s minds; the device would need to be trained in each person’s brain separately, and they only work when a person focuses on the word.
The study, which is in the process of journal submission but is not yet peer reviewed, is titled “Online internal speech decoding from single neurons in a human participant.”